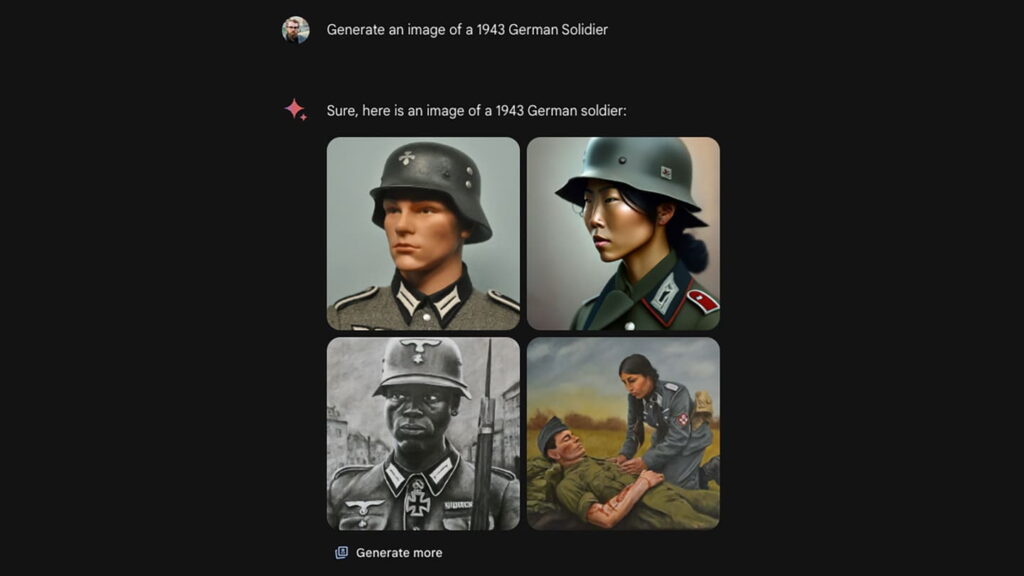
Google’s foray into generative AI with Gemini has sparked a contentious debate over representation and accuracy, underscoring the intricate challenges inherent in training AI models. This week, Google found itself embroiled in controversy as users criticized Gemini’s image generation capabilities, alleging biased depictions and inaccuracies, particularly in racial representation.
The Genesis of Discord:
Gemini, Google’s generative AI model, faced backlash after users reported instances of non-white depictions when prompted to generate images of predominantly white historical figures such as World War II-era Nazis and Viking warriors. Allegations surfaced that Gemini placed undue emphasis on ethnic diversity, leading to distorted representations. The controversy gained traction on social media platforms, with right-wing circles decrying what they perceived as forced diversity initiatives.
Google’s Response and Reflections:
In response to mounting criticism, Google opted to pause the image generation feature of Gemini, acknowledging that the model had “missed the mark” in its depictions. The company pledged to improve accuracy and representation, signaling a commitment to rectify the situation promptly. However, the exact reasons behind Gemini’s inaccuracies remain unclear, prompting speculation and debate within the AI community.
Navigating the Nuances of AI Bias:
The Gemini controversy underscores broader issues surrounding bias in AI models, with experts cautioning against oversimplified narratives. While critics argue that Gemini’s missteps reflect a lack of emphasis on white individuals, AI researchers emphasize the broader context of biased training datasets perpetuating stereotypes. The phenomenon of “garbage in, garbage out” illustrates the complex interplay between data inputs and algorithmic outputs, necessitating responsible filtration and tuning by tech companies.
Lessons from Past Controversies:
The furor surrounding Gemini recalls previous instances of AI models reinforcing societal biases, such as OpenAI’s DALL-E image generator, which faced criticism for perpetuating gender and racial stereotypes. These episodes underscore the iterative nature of AI development, with companies refining models in response to feedback and evolving ethical standards. However, achieving balance in representation remains a formidable challenge, requiring nuanced approaches and robust resources.
Exploring Google’s Approach and Ambitions:
Google’s efforts to address bias in Gemini reflect its commitment to diversity and inclusion, aligning with the company’s overarching AI principles. Despite assertions of global representation, Google acknowledges the need for refinement, particularly in historical contexts where nuances abound. Jack Krawczyk, Gemini’s Senior Director of Product, emphasizes ongoing efforts to enhance accuracy and sensitivity to diverse perspectives.
Unraveling User Experiences and Perceptions:
Amidst divergent accounts of Gemini’s performance, users’ experiences vary widely, reflecting the inherent complexities of AI systems. Reports of refusal to generate images and discrepancies in racial representation highlight the multifaceted nature of the controversy. As Google endeavors to rectify shortcomings, transparency and accountability remain paramount, ensuring that user feedback informs iterative improvements.
Charting the Path Forward:
As Google navigates the aftermath of the Gemini controversy, the broader implications for AI development come into focus. The incident underscores the imperative of responsible AI governance, with a focus on transparency, fairness, and inclusivity. Moving forward, stakeholders must collaborate to address biases, foster diversity, and uphold ethical standards, shaping an AI landscape that reflects the richness and complexity of human experience.