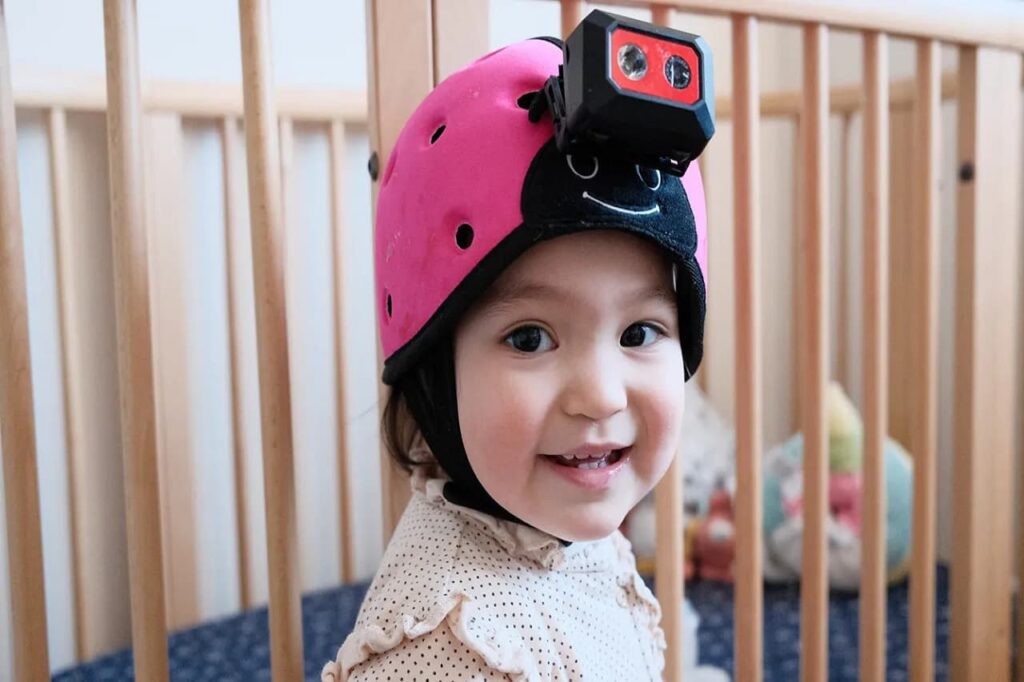
In an exciting new development, artificial intelligence researchers have shown that AI can learn to associate words with objects in much the same way human toddlers do—by observing the world around them. Using a unique approach, a team from New York University’s Center for Data Science trained an AI model using over 60 hours of video and audio data captured by a headcam worn by a toddler named Sam. Their findings, published in Science, could pave the way for more human-like AI learning models in the future.
AI Learning Like a Toddler
Children typically begin to learn their first words between 6 to 9 months of age, and by the time they turn two, they usually have a vocabulary of around 300 words. However, how exactly children associate words with objects remains a mystery. To explore this process, researchers decided to simulate the learning experience of a child using an AI model.
Sam, the toddler at the center of the study, wore a light headcam for 19 months, from age six months to two years. The camera captured over 600,000 video frames and 37,500 transcribed utterances from people around him. The data revealed a vivid picture of a child’s daily life, capturing moments of eating, playing, and interacting with the world. This natural, real-world data became the foundation for training the AI model.
Training the AI Model: No External Labels, Just Associations
Rather than relying on pre-labeled data, the researchers used a self-supervised model, allowing the AI to learn directly from the visual and auditory experiences captured by the headcam. The model had two modules: one analyzed the video frames, while the other processed transcribed speech. The goal was to mimic how a child learns by associating words with the objects they see and hear in context.
The results were promising. When researchers tested the AI, they showed it four images and asked it to match the correct one to a given word, such as “ball,” “crib,” or “tree.” The model successfully identified the correct image 61.6% of the time, which was comparable to AI models trained on much larger datasets. Even more impressive, the toddler-trained AI was able to identify objects that were not included in its training data, suggesting that the model could generalize its learning to new scenarios.
Insights into How Children Learn
This research provides valuable insights into how children might learn language. The AI’s ability to make correct associations from limited data suggests that, like children, AI can acquire vocabulary simply through observing the world and making connections between words and visuals. As NYU professor Brenden Lake, co-author of the study, explained, “It seems we can get more with just learning than commonly thought.”
By studying how children learn through exposure to natural language, the study could inform future AI development. Traditional machine learning models require vast amounts of data to function, often pulled from massive datasets that are costly and may introduce biases. In contrast, this research suggests that AI can learn much more efficiently and naturally by mimicking the human learning process, without relying on huge amounts of labeled data.
The Future of AI Learning
This study hints at a potential shift in how AI models might be developed in the future. Rather than depending on vast quantities of pre-labeled data, AI could be trained to learn more like humans—through observation and interaction with the world. The findings challenge the traditional approach to AI development, suggesting that learning from naturalistic data, like a toddler’s experiences, could be just as effective.
As the field of AI continues to evolve, these insights could lead to more adaptive and efficient systems, capable of learning and understanding language in ways similar to how children acquire their first words. For future AI researchers and developers, this approach might offer a valuable framework for creating smarter, more human-like models.