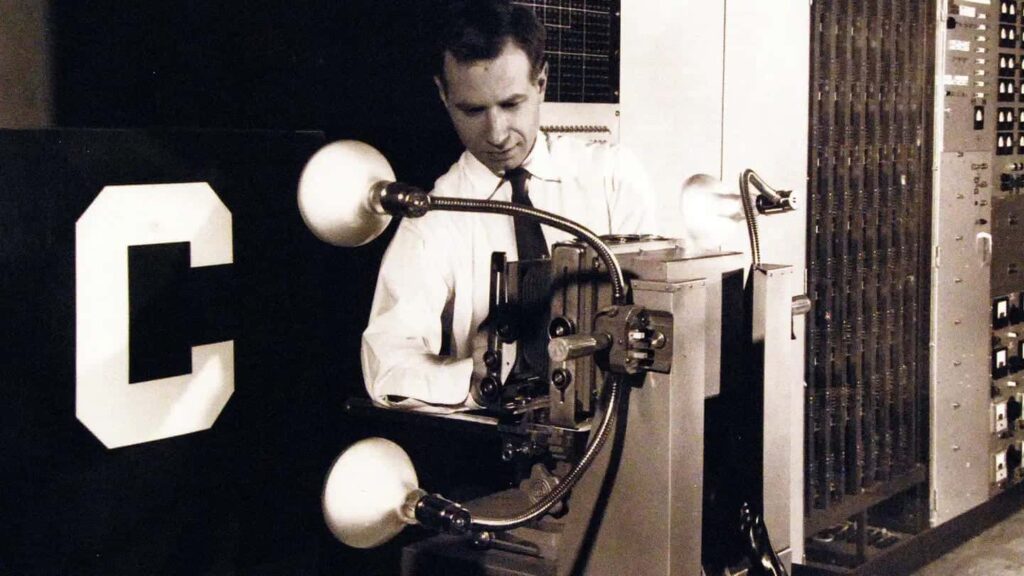
Back in 1958, a groundbreaking innovation in the form of the Perceptron, a room-sized computer, was unveiled, sparking dreams of machines possessing human-like abilities. This event, mentioned in a modest article buried within The New York Times, heralded the potential for machines capable of walking, talking, seeing, writing, reproducing, and even attaining consciousness, as envisioned by the U.S. Navy. Over sixty years later, similar aspirations persist in the realm of contemporary artificial intelligence (AI). But what has truly changed since then?
The journey of AI has been characterized by cycles of enthusiasm and disappointment, akin to a rollercoaster ride through the decades. While today’s AI landscape appears buoyed by optimism and rapid advancement, it behooves us to heed the lessons of history and recognize the recurring patterns of boom and bust.
The Perceptron, credited to Frank Rosenblatt, marked a seminal moment in AI history, laying the groundwork for subsequent developments. Functioning as a learning machine, it aimed to discern whether an image belonged to one of two categories, adapting its connections based on feedback—a process reminiscent of contemporary machine learning algorithms. Fast forward to the present, and we witness the evolution of AI through sophisticated neural networks like ChatGPT and DALL-E, exhibiting enhanced capabilities albeit rooted in the same principles as the Perceptron.
Following the initial excitement surrounding the Perceptron, lofty predictions emerged, envisioning machines with human-like intelligence by the 1970s. However, reality fell short of these expectations, leading to a period of disillusionment known as the first AI winter. Despite subsequent resurgences in the 1980s, epitomized by the advent of expert systems and promising applications across various domains, the field once again encountered setbacks, entering a second AI winter marked by the limitations of existing technologies.
The 1990s witnessed a paradigm shift in AI research, characterized by a shift towards data-driven approaches and the resurgence of neural networks in a digital landscape. This era heralded significant advancements, facilitated by the proliferation of the internet and enhanced computing capabilities, laying the groundwork for modern AI applications.
Today, as confidence in AI reaches new heights, echoes of past promises resound. Phrases like “artificial general intelligence” (AGI) evoke visions of machines on par with human cognition, capable of self-awareness, problem-solving, learning, and possibly even consciousness. Yet, amidst the optimism, it is imperative to acknowledge the persistent challenges that have plagued AI throughout its history.
The perennial “knowledge problem” persists, manifesting in the inability of AI systems to grasp nuances of language and context, evident in their struggles with idioms, metaphors, and sarcasm. Despite advancements in object recognition, AI’s susceptibility to misinterpretation underscores the lingering gap between artificial and human intelligence.
Indeed, AI’s susceptibility to manipulation underscores the need for caution and critical reflection. While contemporary AI may bear little resemblance to its predecessors, the fundamental challenges endure. History serves as a poignant reminder that progress is often accompanied by pitfalls, urging us to approach the promises of AI with tempered optimism and a keen awareness of the past. As we navigate the ever-evolving landscape of artificial intelligence, the lessons of history serve as beacons, guiding our path forward.